How Large Companies Can Grow Their Data and Analytics Talent
Companies need to identify the type of talent they need in order to become data-driven.
Topics
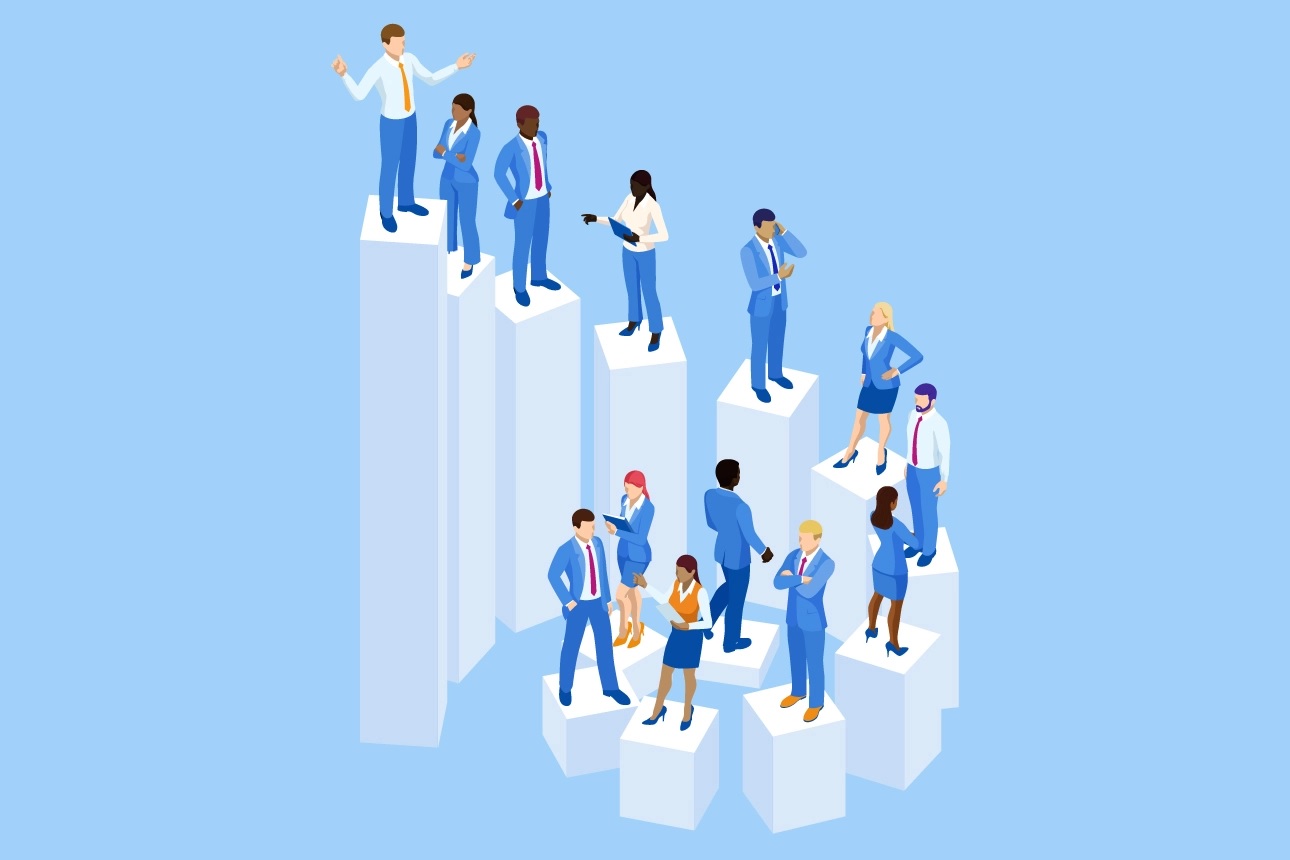
While many companies are hiring data scientists and other types of analytical and artificial intelligence talent, there is little consensus within and across companies about the qualifications for such roles. The term data scientist might mean a job with a heavy emphasis on statistics, open-source coding, or working with executives to solve business problems with data and analysis. The idea of data scientist “unicorns” who possess all these skills at high levels was never very realistic.
As the job has grown more popular and sought-after, an increasing number of professionals have begun to use it to describe their role. Colleges and universities have responded to the demand as well by offering hundreds of new programs on data science and analytics. But the skills taught in such programs vary widely, and some universities offer multiple programs with different emphases. For both newly hired and experienced employees, titles such as data scientist and quantitative analyst are not likely to be good guides to their actual capabilities.
While there are initiatives to standardize the different types of data and analytics roles and requisite skills across organizations, they are in the early stages. The idea behind these initiatives is excellent, but developing new standards typically takes many years.
In the meantime, companies need to devote considerable attention to classifying and certifying the different types of analytical jobs they have and need. They also would benefit from expanding their talent pools by working with universities directly on educational programs and by building and nurturing communities within their organizations to develop employees for their data teams. These steps are essential for companies looking to use analytics to improve both operations and opportunities for digital innovation — companies such as TD Bank Group.
The idea of data scientist ‘unicorns’ who possess all these skills at high levels was never very realistic.
Understand the Data Roles Needed
Analytics and AI talent is a scarce and valuable resource for every company, but particularly for those with a desire to be data-driven. That’s the situation at TD Bank Group, a large North American bank (the largest by assets in Canada). TD made the decision to use its vast amounts of data to power new data-driven customer experiences and improve its own processes. To do so, it has embraced data, analytics, AI — and the need to nurture the talent that will use those tools throughout the organization.
TD executives made a series of internal investments directed at the critical importance of assessing, hiring, and motivating its data and analytics talent. Peter Husar, vice president of enterprise analytics strategy and planning, told me that he and his colleagues saw an opportunity to expand the role of analytics by first bringing consistency to the job definitions and positions related to data and
analytics that existed across the bank.
Husar and his team focused on understanding who was in their data and analytics community in order to provide a clear picture of their talent landscape. They worked quickly to create standardized job groupings and classifications by identifying seven job families (roles that focused on advanced analytics, business application management, information management, insights, business intelligence and reporting, data governance, and visualization).
Within each job family, specific jobs were defined in detail — in all, 65 different roles across seven job families. For each role, several attributes were described, including its primary purpose, its key accountabilities, its breadth and depth, and the experience and education required to perform it. Individual contributor attributes were identified, as well as attributes for people managers. Additional
competencies were labeled across the job classifications, along with competency assessments and a self-assessment process. With this new framework, TD employees working in data and analytics roles were then mapped to the job families and specific jobs. TD has an Office of the Chief Data Officer, which oversees enterprise data management, analytics, and AI.
Although most companies don’t have the scale and scope of analytical talent that TD does, many medium to large companies have some data scientists and quantitative analysts. In order for their projects to succeed, it’s important to create at least a simple classification of their capabilities. Are they primarily oriented to statistical analysis and modeling, coding IT solutions, or translating business issues into data and analytics solutions? Projects may require all three skills, but those skills are unlikely to be found in one person.
Acquire and Retain New Talent
In addition to more effectively managing existing data and analytics talent, organizations need to make a concerted effort to bring on and retain highly skilled employees.
In 2018, TD acquired artificial intelligence company Layer 6 and made one of its cofounders, Tomi Poutanen, the chief AI officer for the bank. TD continues to transfer Layer 6’s capabilities to the bank at large — including information-sharing programs at Layer 6 for data and analytics employees.
The bank has built strong relationships with a number of Canadian universities, including the University of Toronto’s Rotman School of Management, Western University, Queen’s University, and York University’s Schulich School of Business. TD sponsors events at these institutions, including hackathons and speaker series. At Rotman, TD has funded both the TD Management Data and
Analytics Lab and the Rotman Financial Innovation Hub in Advanced Analytics to help foster the development of data and analytics professionals.
TD also works closely with leading AI research programs in Canada, including those at the Vector Institute in Toronto, of which TD was a founding sponsor, and Mila in Montreal, in order to foster partnerships and connections.
These efforts have not gone unnoticed. TD has been rated among the most attractive employers for data and analytics talent by Canadian students: In a large student survey in Canada, analytics students ranked TD as one of the top four companies they would consider working for.
Most organizations will have to turn to multiple suppliers of talent to acquire the range of skills needed. They should work with the universities and colleges that are providing them with new talent to better understand the foci of the schools’ data science and analytics programs. They should also embark on using job standardization and classification to design internal education and skills development programs. When organizations make clear which skills are associated with which jobs, employees can more easily decide which roles they want and acquire the skills to perform them.
Build a Data and Analytics Community
TD data and analytics leaders are conscious of the need to build community and knowledge-sharing approaches among the data and analytics professionals across the bank. That includes providing opportunities for data- and analytics-oriented employees to network and hear about trends in the industry. Five years ago, the bank had its first annual Big Data & Analytics Summit, with 40 employees attending. In 2019, the bank had its fifth annual summit (disclosure: I was a paid speaker at this event), with over 2,000 employees in attendance — and the numbers continue to grow.
Another key aspect of community is volunteering. An initiative called TD Mindpower: Analytics for Social Good was created in 2019 to pair data and analytics employees with data and analytics projects for nonprofits in Canada and the U.S. As part of the bank’s acquisition of Layer 6, a pledge was also made to dedicate time and talent to work specifically on AI projects for social good.
TD’s initial job-mapping exercise led to a clearer vision for how individuals can move around, upskill, and grow their career paths. The team spearheading the plan created educational materials to help data and analytics employees develop their skills and made those materials available on an enterprisewide online platform for self-learning and development. The bank is now focused on expanding an understanding of the potential of data and analytics to all TD employees and has developed learning curricula for nonanalytics professionals as well. The goal is to further embed data-driven decision-making in all aspects of the bank.
According to Husar, data, analytics, and AI at TD have come a long way in the past five years and are helping to shape and deliver tangible results for customers and employees. In particular, during these unprecedented times, the bank has shown its ability to pivot, leverage data and analysis, and mobilize quickly to respond to the needs of its customers, spinning up new and innovative ways to stay connected and answer questions. For example, TD has used customer data and analytics to personalize customer communication. Customers who visit often received targeted messages about changes in bank branch hours, and those most likely to have received a stimulus check got messages about how to make mobile deposits.
Whether people are called data scientists, quantitative analysts, or something else, these titles are still relatively new to companies. It’s not surprising that there is little consensus on their meaning. Over time, new titles will emerge that better describe the particular activities involved in a job; data engineer, for example, has already arisen to describe people who spend most of their time wrangling data. Until more consensus is reached across our society, however, it’s important for companies to emulate TD Bank and clearly identify the types of talent required to become data-driven and then acquire it, nurture it, and unleash it.